Rate-distortion theory
In this post, I will summarize Shannon’s rate-distortion theory, including some self-contained definitions and proofs to the converse and direction part of the main theorem. The rate-distortion theorem plays a crucial role in modern signal processing, especially in understanding the limit of data compression. I will try to keep the material short and concise.
Table of Contents
1. Definitions
We have a sequence \(X^n=(X_1, \dots, X_n)\) with \(X_i\) being sampled IID according to a source distribution \(P_X\). We also assume \(X_i\) takes on values from a finite alphabet \(\mathcal{X}\). Let us define an encoder-decoder pair with encoding function \(f_n: \mathcal{X}^n \rightarrow \{1,\dots, 2^{nR}\}\) and decoding function \(\phi_n: \{1,\dots, 2^{nR}\} \rightarrow \hat{\mathcal{X}}^n\), where \(R\) is the rate (denoting bits per source symbol) and \(\hat{\mathcal{X}}\) is the reconstruction alphabet. In plain words, the encoder \(f_n\) maps \(X^n\) to a index of a codebook \(\mathcal{C}\) consisting of \(2^{nR}\) codewords, and the decoder \(\phi_n\) returns the corresponding codeword \(\hat{X}^n\) which is one of the rows in \(\mathcal{C}\).
One example of the scheme above is the representation of real numbers in computers. We would need infinite precision, thus infinite bits, to represent a number in \(\mathbb{R}\), so we instead truncate it to \(32\)-bit floating point, resulting in a codebook consisting \(2^{32}\) possible numbers. Such a scheme is also called quantization. We essentially quantize numbers in \(\mathbb{R}\) using a finite set of rational numbers \(\mathbb{Q}\).
To measure the quality of the chosen scheme, which is a pair \((f_n, \phi_n)\), we can measure the distortion between the input and the reconstruction as \(d(X^n,\hat{X}^n)=\frac{1}{n}\sum_{i=1}^n d(X_i, \hat{X}_i)\). Here, \(d:\mathcal{X}\times \hat{\mathcal{X}} \rightarrow \mathbb{R}^+\) is the distortion measure. Some popular choices of distortion measure includes:
- Hamming distortion that is given by \(d(x,\hat{x}) = [x \neq \hat{x}]\). Here, \([\cdot]\) denotes the indicator function.
- Squared-error distortion that is given by \(d(x,\hat{x})=(x-\hat{x})^2\).
For simplicity, I assume that Hamming distortion is used in this post. Moving on, the following introduces several definitions highlighting the interplay between rate \(R\) and distortion \(D\).
Definition 1. The rate distortion pair \((R,D)\) is achievable if there exists a sequence of \((f_n,\phi_n)\) with \(\lim_{n\rightarrow \infty} \mathbb{E} d(X^n, \phi_n(f_n(X^n))) \leq D\).
Definition 2. The rate distortion region is the closure of all achievable \((R,D)\).
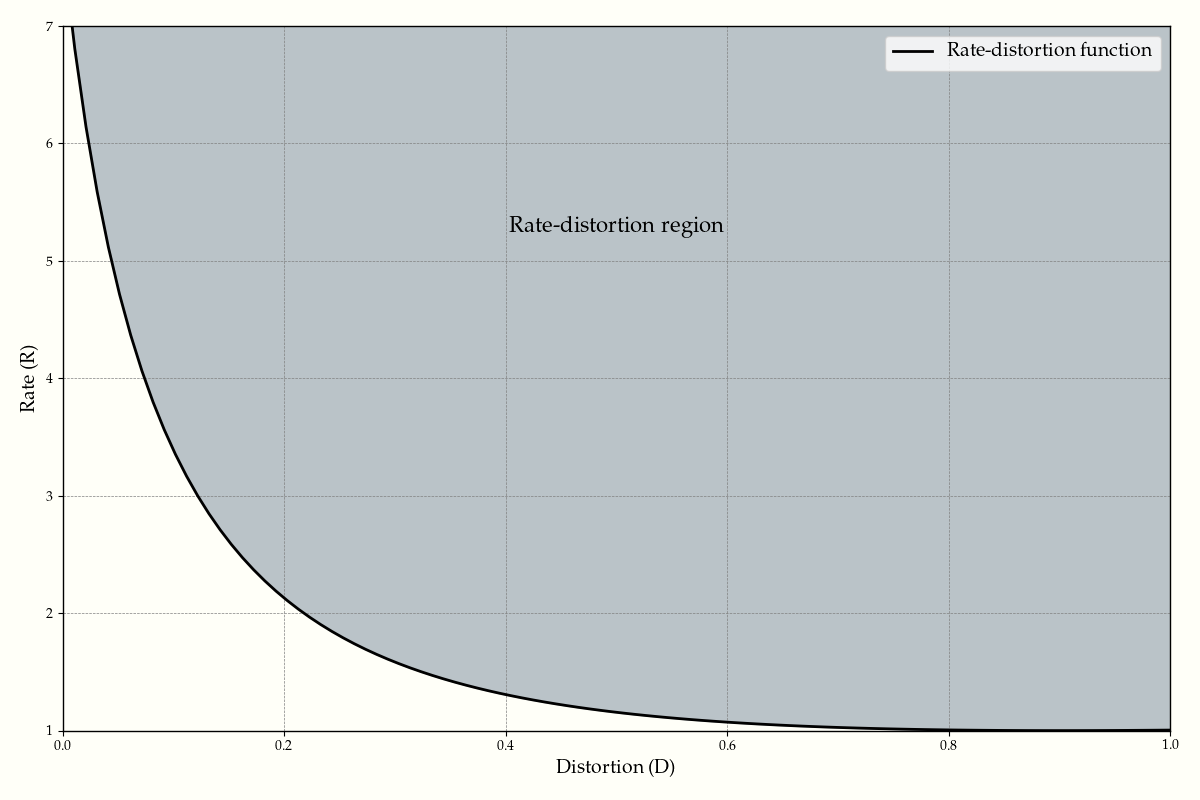
Definition 3. The rate distortion function \(R(D)\) is the infimum over all rates \(R\) such that \((R,D)\) is achievable for a given distortion \(D\).
Definition 4. The information rate distortion function is defined as
\[R^{(I)}(D) = \underset{P_{\hat{X}|X}:\sum_{x,\hat{x}} P(x)P(\hat{x}|x)d(x, \hat{x})\leq D}{\min} I(X;\hat{X})\]Where \(I(\cdot;\cdot)\) is the mutual information function and the minimum is taken over all possible \(P_{\hat{X}|X}\), assuming that the source distribution \(P_X\) is fixed. Alternatively, we can also define \(R^{(I)}(D)\) as the minimization over the joint \(P_{\hat{X}X}\) as long as the marginal matches the given \(P_X\).
2. The rate-distortion theorem
In his foundational work in information theory, Claude Shannon stated a key result in rate-distortion theory:
Theorem 1.
\[R(D)=R^{(I)}(D)=\underset{P_{\hat{X}|X}:\sum_{x,\hat{x}} P(x)P(\hat{x}|x)d(x, \hat{x})\leq D}{\min} I(X;\hat{X})\]This gives the original definition of rate distortion function \(R(D)\) an operational value as an optimization problem. Spelling things out, the theorem has two parts:
- Direct part: If \(R>R^{(I)}(D)\) then \((R,D)\) is achievable.
- Converse part: If \((R,D)\) is achievable then \(R\geq R^{(I)}(D)\).
2.1. Converse part
The proof for the converse is rather straighforward and depends only on some properties of \(R^{(I)}(D)\), namely \(R^{(I)}(D)\) is monotonically non-increasing, convex, and continuous. For interested readers, you can refer to the proofs of these properties in Elements of Information Theory by Thomas & Cover.
Claim 1. \(nR \geq I(X^n; \hat{X}^n)\)
Proof of claim 1.
\[\begin{align} I(X^n; \hat{X}^n) &\leq I(X^n; f_n(X^n)) &&\text{(Data processing ineq.)}\\ &= H(f_n(X^n)) &&(H(f_n(X^n)|X^n)=0)\\ &\leq nR &&(\text{Codebook is of size } 2^{nR}) \end{align}\]Proof of the converse part.
\[\begin{align} nR &\geq I(X^n; \hat{X}^n) &&\text{(Claim 1)}\\ &= H(X^n) - H(X^n|\hat{X}^n)\\ &= \sum_i H(X_i) - \sum_i H(X_i|X^{i-1}, \hat{X}^n) &&\text{(Chain rule)}\\ &\geq \sum_i H(X_i) - \sum_i H(X_i|X^{i-1}) &&\text{(Conditioning reduces entropy)}\\ &= \sum_i I(X_i; \hat{X}_i)\\ &\geq \sum_i R^{(I)} (\mathbb{E} d(X_i, \hat{X}_i)) &&(\text{By def. of } R^{(I)}(D))\\ &= n \sum_i \frac{1}{n} R^{(I)} (\mathbb{E} d(X_i, \hat{X}_i))\\ &\geq n R^{(I)} \left(\frac{1}{n} \sum_i \mathbb{E} d(X_i, \hat{X}_i) \right) &&(\text{Convexity of } R^{(I)}(D) \text{ and Jensen's ineq.})\\ &= n R^{(I)} (\mathbb{E} d(X^n, \hat{X}^n)) &&\text{(By def. of distortion)}\\ &\geq n R^{(I)} (D) &&(\text{Monotonicity of } R^{(I)}(D) \text{ and the claim that } \mathbb{E} d(X^n, \hat{X}^n) \leq D)\\ \end{align}\]The above completes the proof of the converse part. Intuitively, the converse says that if you give me a wonderful scheme that achieves distortion \(\leq D\), I will show that your rate \(R\) must be at least \(R^{(I)}(D)\).
2.2. Direct part
In contrast to the converse part, the proof for the direct part is tricky. Nevertheless, it is actually quite similar to the direct part of noise-channel coding theorem. The crux of this proof would follow the random codebook construction with some small differences.
I will start by laying out some ingredients for the main proof.
Lemma 1. Given \(N\) IID Bernoulli \(p\) variables \(X_1,\dots,X_n\), we have that
\[P(\text{at least 1 success}) = P((X_1=1) \lor \cdots \lor (X_n=1)) \rightarrow 1 \text{ if } np\rightarrow \infty\]Proof of lemma 1.
\[\begin{align} P(\text{at least 1 success}) &= 1-P(\text{failure in all trials}) \\ &\geq 1-(1-p)^n \\ &\geq 1-\exp(-np) \rightarrow 1 \text{ as } np\rightarrow \infty \end{align}\]Lemma 2. Given a function \(g:\mathcal{X}\rightarrow \mathbb{R}^+\) and a sequence \(\underline{x}=(x_1,\dots,x_n)\) with \(x_i\in\mathcal{X}\), the following holds:
\[\underline{x} \in \mathcal{T}_{\varepsilon}^{(n)} (P_X)\Rightarrow \frac{1}{n} \sum_i g(x_i) \leq (1+\varepsilon) \mathbb{E}g(X)\]Here, \(\mathcal{T}_{\varepsilon}^{(n)}(P_X)\) is a strongly typical set with tolerence \(\varepsilon\).
Proof of lemma 2. The proof follows naturally from the definition of a strongly typical set.
Lemma 3. Given two sequences of random variables \(\{\tilde{X}_i\}, \{\tilde{Y}_i\}\) that are drawn IID from \(P_X\) and \(P_Y\) respectively, where \(P_X\) and \(P_Y\) are the marginals of some \(P_{XY}\), then
\[Pr((\tilde{X}, \tilde{Y})\in \mathcal{A}_{\varepsilon}^{(n)}(P_{XY})) \leq 2^{-n(I(X;Y)-3\varepsilon)}\]Where \(\mathcal{A}_{\varepsilon}^{(n)}(P_{XY})\) is a weakly typical set with tolerence $\varepsilon$.
Lemma 4. Given \(0<\varepsilon'<\varepsilon\), if \(\underline{x}\in \mathcal{T}_{\varepsilon'}^{(n)}(P_X)\) and \(\{Y_i\} ~\) IID from \(P_Y\) then:
\[Pr((\underline{x}, \underline{Y})\in \mathcal{T}_{\varepsilon}^{(n)}(P_{XY})) \geq 2^{-n(I(X;Y)+4\delta_{XY})}\]The lemma 3 has been proven in the previous blog post, and the proof of lemma 4 is omitted since it is quite complicated.
Proof of the direct part: Given the source distribution \(P_X\), we fix the conditional probability \(P_{\hat{X}|X}\) such that it satisfies \(\mathbb{E}_{P_{X\hat{X}}} d(X,\hat{X}) \leq D\). We will show that if \(R > I_{P_{X\hat{X}}}(X;\hat{X}) + \tilde{\epsilon}\) then \((R,D)\) is achievable. If we are able to prove this, the proof in the case \(R>R^{(I)}(D)\) would follow naturally.
Since we already have \(P_X\) and \(P_{\hat{X}\vert X}\), we also get the output symbol probability \(P_\hat{X}\) using the law of total probability:
\[P_{\hat{X}}(\hat{x}) = \sum_{x} P(\hat{x}|x) P(x)\]Similar to the direct part of noisy-channel coding, we consider a random codebook \(\mathcal{C}\in \mathcal{\hat{X}}^{2^{nR}\times n}\) where the row elements follow \(P_{\hat{X}}\). In this proof, the decoder follows the strongly typical decoding scheme; given a source sequence \(\underline{x}\in \mathcal{X}^n\), we search for the existence of a row \(j\) in \(\mathcal{C}\) such that \((\underline{x}, \hat{x}(j))\in \mathcal{T}_{\epsilon}^{(n)}(P_X \circ P_{\hat{X}|X})\). Also, to be more precise with the constant terms invovled, we assume that \(0<\epsilon'<\epsilon<\tilde{\epsilon}\). We define the event of “success” for the decoder \(\phi_n\) if there exists such \(j\) satisfying the above statement, and if so, the reconstruction for \(\underline{x}\) is then \(\hat{x}(j)\) (the row \(j\) in \(\mathcal{C}\)). In this case, the distortion is upper bounded by:
\[\begin{align} d(\underline{x}, \hat{x}(j)) &\leq \mathbb{E}[d(x,\hat{x})](1+\epsilon) &\text{(Lemma 2)}\\ &=D(1+\epsilon) &\text{(By assumption)} \end{align}\]In the case where there is no such \(j\) exist, the distortion is assumed to be at most \(d_\max \doteq \underset{x,\hat{x}}{\max} d(x,\hat{x})\). For this, the expected distortion, assuming that \(\underline{x}, \mathcal{C}\) are random, is upper bounded by:
\[\mathbb{E}[d(X,\hat{X})] \leq \text{Pr(success)}D(1+\epsilon) + \text{Pr(failure)} d_\max\]For a fixed codebook \(\mathcal{C}\),
\[\mathbb{E}[d(X,\hat{X})\vert \mathcal{C}] \leq \text{Pr}(\text{success}\vert \mathcal{C})D(1+\epsilon) + \text{Pr}(\text{failure}\vert \mathcal{C}) d_\max\]The plan now is quite similar to the noisy-channel coding’s proof: if I can upper bound the expected distortion \(\mathbb{E}[d(X,\hat{X})]\) (averaged over \(\mathcal{C}\)) by some amount \(\gamma\), then there must exist a codebook \(\mathcal{C}^*\) that actually achieves the distortion of at most \(\gamma\). To achieve this plan, we shall turn our attention to \(\text{Pr}(\text{success})\) which we can eventually show to happen with almost guarantee. There are two ways we can factor \(\text{Pr}(\text{success})\) with the law of total probability:
\[\begin{align} \text{Pr}(\text{success}) &= \sum_{\mathcal{C}} \text{Pr}(\mathcal{C}) \text{Pr}(\text{success} | \mathcal{C}) &\text{(1)}\\ \text{Pr}(\text{success}) &= \sum_{\underline{x}} \text{Pr}(\underline{x}) \text{Pr}(\text{success} | \underline{x}) &\text{(2)} \end{align}\]Notice that the right-hand side of \((2)\) depends on the choice of \(\mathcal{C}\). The important point is that we are effectively recasting the randomness of \(\mathcal{C}\) to the randomness of \(\mathcal{X}\), which eases the analysis. We then have the following decomposition:
\[\begin{align} \text{Pr}(\text{success}) &= \sum_{\xi \in \mathcal{T}_{\epsilon'}^{(n)}(P_X)} P(\underline{X}=\xi) P(\text{success} | \underline{X}=\xi) + \sum_{\xi \notin \mathcal{T}_{\epsilon'}^{(n)}(P_X)} P(\underline{X}=\xi) P(\text{success} | \underline{X}=\xi)\\ &\geq \sum_{\xi \in \mathcal{T}_{\epsilon'}^{(n)}(P_X)} P(\underline{X}=\xi) P(\text{success} | \underline{X}=\xi) \end{align}\]From lemma 1 and 4, we know that:
\[\begin{align} P(\underline{X}=\xi) P(\text{success} | \underline{X}=\xi) &\geq 1- \exp(-2^{nR}2^{-n(I(X;\hat{X})+4\delta_{X\hat{X}})})\\ &= 1- \exp(-2^{-n(I(X;\hat{X})+4\delta_{X\hat{X}}-R)}) \end{align}\]Thus,
\[\begin{align} \text{Pr}(\text{success}) &\geq \sum_{\xi \in \mathcal{T}_{\epsilon'}^{(n)}(P_X)} P(\underline{X}=\xi) 1-\exp(-2^{-n(I(X;\hat{X})+4\delta_{X\hat{X}}-R)})\\ &= 1- \exp(-2^{-n(I(X;\hat{X})+4\delta_{X\hat{X}}-R)}) \end{align}\]If \(R > I(X;\hat{X})+4\delta_{X\hat{X}}\), we can drive \(\text{Pr}(\text{success})\rightarrow 1\) for some large \(n\). Consequently, this tells us that if \(R > I(X;\hat{X})+4\delta_{X\hat{X}}\) then \(\mathbb{E}[d(X,\hat{X})]\leq D(1+\epsilon)\), implying the existence of codebook \(\mathcal{C}^*\) whose distortion is at most \(D(1+\epsilon)\). To get the prove for \(R>R^{(I)}(D)\), we just need to set \(P_{\hat{X}\vert X}\) to the minimizer of the functional \(R^{(I)}(D)\), and set \(\delta_{X\hat{X}}=\frac{\tilde{\epsilon}}{4}\).